Proceedings Vol. 26 (2020)
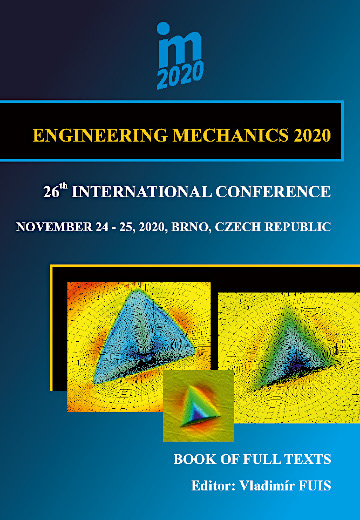
ENGINEERING MECHANICS 2020
November 24 – 25, 2020, Brno, Czech Republic
Copyright © 2020 Brno University of Technology Institute of Solid Mechanics, Mechatronics and Biomechanics
ISSN 1805-8248 (printed)
ISSN 1805-8256 (electronic)
list of papers scientific commitee
pages 274 - 277, full text

Recently there is an increasing endeavour to take into account the underlying uncertainties by stochastic modelling in order to make the numerical predictions as realistic as possible. Uncertainty quantification deals with distinct sources of nondeterminism. A lack of knowledge is expressed by epistemic uncertainties while aleatory uncertainties formulate an inherent randomness. In the case of estimating aleatory uncertainty, the task is to infer unknown but fixed probability density function and the corresponding epistemic uncertainty about this estimation. In order to avoid too strict assumptions about the unknown density function (e.g. prescription of a specific parameterised family of probability density functions), it can be modelled hierarchically by a stochastic process via the Bayesian nonparametric approach. The contribution presents application of a Dirichlet process mixture in modelling the aleatory uncertainty.
back to list of papers
Ownership of copyright in original research articles remains with the Authors, and provided that, when reproducing parts of the contribution, the Authors acknowledge and/or reference the Proceedings, the Authors do not need to seek permission for re-use of their material.
All papers were reviewed by members of the scientific committee.