Proceedings Vol. 26 (2020)
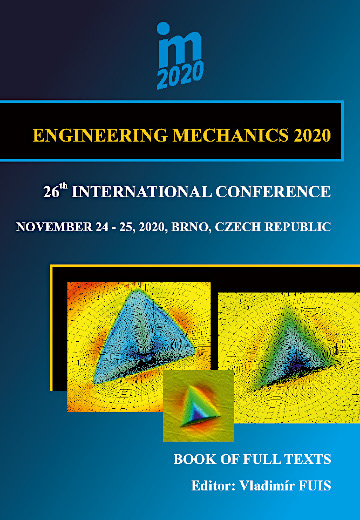
ENGINEERING MECHANICS 2020
November 24 – 25, 2020, Brno, Czech Republic
Copyright © 2020 Brno University of Technology Institute of Solid Mechanics, Mechatronics and Biomechanics
ISSN 1805-8248 (printed)
ISSN 1805-8256 (electronic)
list of papers scientific commitee
pages 428 - 431, full text

The paper deals with the replacement of the analogy PID stroke controller of a bellows pneumatic spring, by machine learning algorithms, specifically deep reinforcement learning. The Deep Deterministic Policy Gradient (DDPG) algorithm used consists of an environment, in this case a pneumatic spring, and an agent which, based on observations of environment, performs actions that lead to the cumulative reward it seeks to maximize. DDPG falls into the category of actor-critic algorithms. It combines the benefits of Q-learning and optimization of a deterministic strategy. Q-learning is represented here in the form of critic, while optimization of strategy is represented in the form of an actor that directly maps the state of the environment to actions. Both the critic and the actor are represented in deep reinforcement learning by deep neural networks. Both of these networks have a target variant of themselves. These target networks are designed to increase the stability and speed of the learning process. The DDPG algorithm also uses a replay buffer, from which the data from which the agent learns is taken in batches.
back to list of papers
Ownership of copyright in original research articles remains with the Authors, and provided that, when reproducing parts of the contribution, the Authors acknowledge and/or reference the Proceedings, the Authors do not need to seek permission for re-use of their material.
All papers were reviewed by members of the scientific committee.